This is the third of four posts on Generative AI:
- Generative AI and large language models: background and contexts
- Generative AI, scholarly and cultural language models, and the return of content
- Generative AI and libraries: 7 contexts
- Generative AI and library services: some directions
It is now a year since the momentous appearance of ChatGPT. So much has happened in that time. Whether one measures by new product and feature announcements, business churn (investment, startups), or policy, safety and ethical debate. Usage is increasingly integrated into daily applications. Much of this has become routine, some of it is tedious, and much still has the ability to surprise. Capacities continue to expand. See the recent inclusion of voice and image capabilities into ChatGPT for example, or the introduction of the confusingly named GPTs, which allow you to create and share custom versions of ChatGPT based on your own data (more below and NYT coverage here).
The recent White House Executive Order on Safe, Secure, and Trustworthy Artificial Intelligence highlighted safety concerns, will influence developments in the US, and has prompted much debate about the role of government, industry and others. Among many announcements, it proposes that government communications be water-marked to assure readers that they are authentic. Of particular interest here is its education directive.
To help ensure the responsible development and deployment of AI in the education sector, the Secretary of Education shall, within 365 days of the date of this order, develop resources, policies, and guidance regarding AI. // White House
Putting the rapid ChatGPT developments side by side with the Executive Order underlines the complexity of the current environment.
This post transitions from the more general AI discussion in earlier posts to one more focused on libraries, before looking more closely at potential library services and responses in an upcoming fourth post.
Here are some important library concerns that recur at various points:
- Care and empathy for library workers faced with more uncertainty.
- New research skills
- Managing potential harmful effects.
- Informed consumer/customer
- Education and awareness for library users and library workers
- Copyrights and fair use
- Advocacy for researcher and learner interest in policy and governance forums
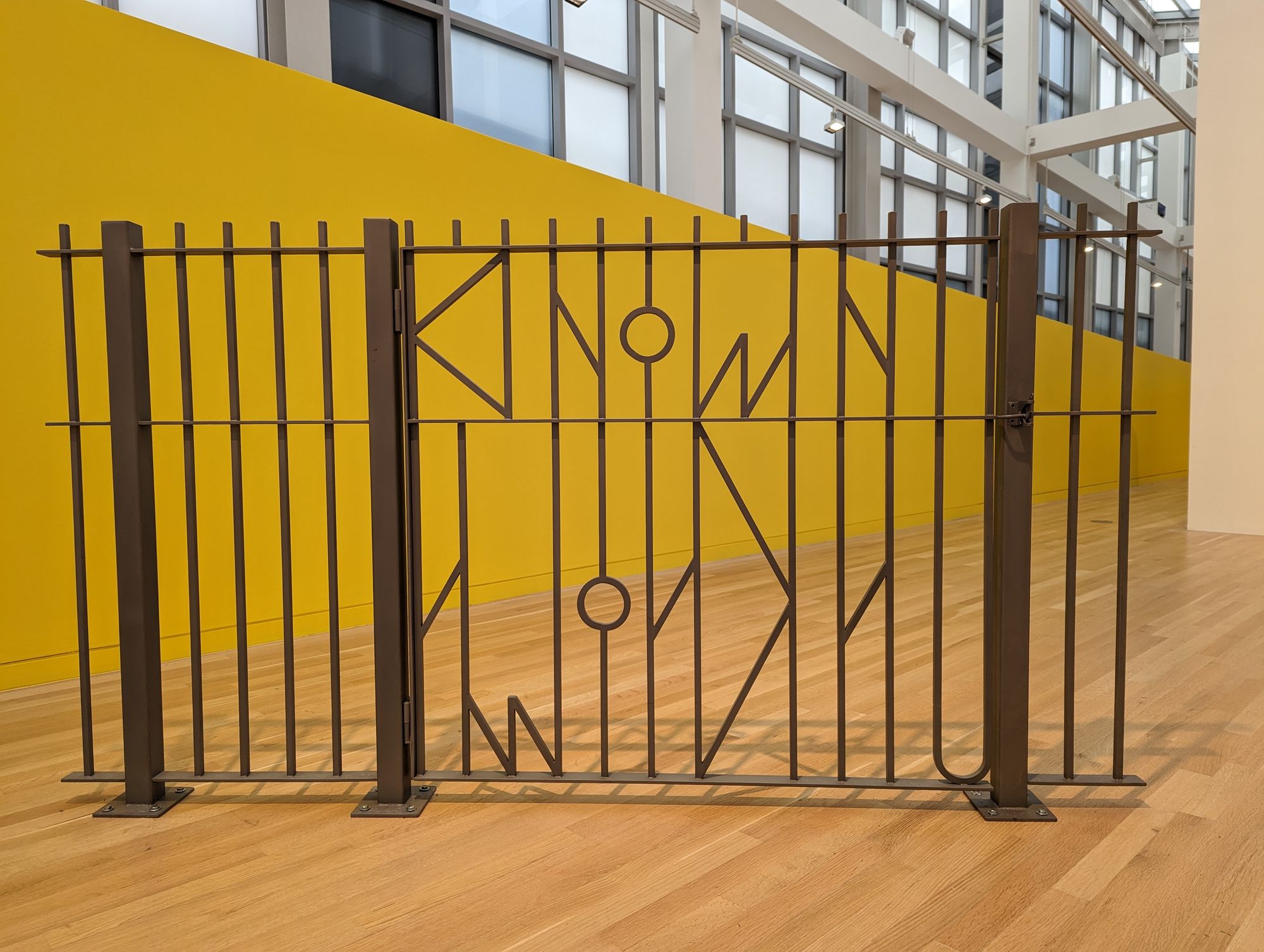
Click for a short list of capacities and issues of importance for libraries in the AI Background Post
① AI is both constructive and problematic
I like this phrase used by scholars Barrett and Orlikowski as they discuss the issues raised by studying technologies at scale: "generating problematic as well as constructive outcomes." As AI goes mainstream (is anything more mainstream than Microsoft Office?), library workers are increasingly having to make day to day decisions about adoption, advice, and policies. Their stakeholder communities will be in various states of enthusiasm or resistance, interest or apathy, knowledge or learning. As library decision-makers look to position the library as a source of advice and expertise, as they make procurement decisions, as they plan for the future, and as they communicate and work with colleagues, the constructive and the problematic will have to be understood and weighed.
Activity is now marked by qualified engagement and informed caution.
There is serious investment and uptake across science and engineering domains, in healthcare, in the law, and in business applications generally. At the same time, AI tools and techniques are becoming more common throughout research and learning lifecycles, accompanied by much debate and caution. While much of this development is seen as productive, there is also intense discussion and clearer appreciation of the problematic. Including but not limited to the following:
- There is growing understanding of the potential dilution of trust and social confidence as synthesized communication or creative works are indistinguishable from human forms. For example, Microsoft has been criticized for the use of AI-generated content on its new pages, responsible for creating 'false and bizarre' stories. But these missteps are a signal of how we are likely to see much of this type of material generated in this way.
- There is strong debate and extensive legal action around the incorporation of intellectual creations into training data for LLMs without agreement, or indeed despite active opposition.
- In some contexts, outputs may be actually harmful. I was recently pointed at a discussion of an eating disorder helpline that took down a chatbot after it gave weight loss advice.
- There is a recognition of the concentration of economic and cultural power in a small number of providers, and an interest in diversifying the ecosystem or the monopoly power of dominant players.
- There is strong advocacy around real social concerns, including the role of hidden labor in testing and training, the quality and security of jobs and work, the impact on climate and power use.
- There is growing awareness of questions around training data, including the propagation of harmful and historically dominant perspectives, and the occlusion of experiences that are under-represented or marginalized on the web and other sources of training data.
- There is growing awareness of why LLMs hallucinate (see below), what limits this puts on use, and how it might be mitigated.
This pervasiveness - and the constructive and problematic characteristics that accompany it - makes the library response necessarily multi-dimensional. Some areas of attention include the following, which may also be relevant to several of the later contexts:
Library work and workers
Empathy. My sense is that as AI discussion and deployment has evolved, the library conversation is moving to qualified engagement and informed caution. However, this is not to brush past the very real human consequences in terms of uncertainty about futures, possible changing job requirements, the need to learn new skills or to cope with changed demands. This is now happening after several unprecedentedly stressful years for library workers. The cumulative effect of these pressures can be draining, and empathy can be difficult. How much have library cultures changed given the experiences of the last few years? I do not know. However, given where we have been, and where we are going, empathy, education and appropriate transparency about planning and direction are now even more critical in the workplace.
Awareness. We all have an interest in staying informed. However, it is almost impossible to keep on top of all that is happening, let alone to understand implications. Many libraries have set up working groups or other mechanisms to track developments, to make recommendations about adoption, and so on. It might be useful, for example, to have some general talking points which can be used in initial discussions with students and faculty. However, not all libraries have the capacity to do this. In this context, there is a special role for consortia, member associations or others to fill the gap. I have been expecting that some library organization might step into this gap producing a regular news and guidance, with a practical library emphasis. There are now various general news digests and awareness resources which might be a model. Some library organizations have released introductory briefings, useful to provide orientation, but these date quickly and are inevitably rather high level. In any case, it is time to move beyond this to more practical decision-making and awareness support. Perhaps some library organization could fund Nicole Hennig to produce regular briefings, such as these pragmatic and helpful posts: How I use generative AI in my work and Evaluating generative AI tools for purchase: a checklist. In a slightly different context, many libraries are watching the Ithaka S+R project on university adoption of AI with interest.
As I read the article [about prompt engineering], I was reminded of the skills involved in the .... reference interview. Interpreting problems and representing them in ways informed by the particular workings of specific tools is a long-standing library skill.
Advice and expertise: the new research skills
As the library positions itself as a source of advice and expertise about use of AI tools in information and data related tasks, it is important to develop understanding of how LLMs and associated tools work, what tools are available, what they are good for and what their limits are. A non-exhaustive list of topics relevant to new research skills includes:
- ChatBot. How is ChatGPT different from Bing Chat or Perplexity.ai? What is each best for? Is it worth going to the fee-based premium options? If the custom chatbots anticipated by OpenAI begin to appear, it will become important to track niche scholarly, cultural or other resources (what about, potentially, the Martha Stewart chatbot, or the Elinor Ostrom chatbot, or the Wired Magazine chatbot).
- Prompt Engineering. This created great interest broadly, and many in the library community saw it as a potentially important area of library specialization. Effective prompting is now an important research skill and there is much guidance about best practice, including providing examples in the prompt, sketching in context, asking for responses in a particular format or style, suggesting a persona for the response, and so on. More advanced techniques have also been explored, including asking the model to explain its reasoning steps. An important aspect of prompt engineering is its use to mitigate hallucinations and harmful outputs. Ethan Mollick talks through some very pragmatic considerations here, considering both images and text. I link to an interesting article below, where the author argues that a more enduring skill than prompt engineering may be problem formulation. As I read the article, I was reminded of the skills involved in the .... reference interview. Interpreting problems and representing them in ways informed by the particular workings of specific tools is a long-standing library skill. Prompt engineering is evolving and it involves more than initial human input, as I discuss further below.

- LLMs. GPT4 dominates debate, but there are other commercial options (Claude 2, for example) and a variety of open source models. How are these different from each other? Are there differences in terms of transparency, use of data for training, and so on? What alternatives exist for those who do not want to use LLMs which are known to have large amounts of unauthorised material in their training data? What LLMs exist that are focused on scholarly and cultural domains?
- Copyrights. What does it mean for one's work to be harvested for use in training an LLM? What does it mean if one's work is included in one of the known training sets? How can one prevent this? What is the current legal situation? Can one use LLMs that are trained on cleared training data? What are the differences between image generation tools in this regard?
- Tools and techniques. And of course, general advice on relevant AI tools and techniques, as one would advise on others, adding an AI dimension. This may be in digital humanities, teaching and learning, and so on. Here is an example from the University of Arizona: Generate topics for your research paper with ChatGPT [Google doc].
- Images. What are the differences between the various image generation tools, and between those and the general purpose tools like ChatGPT which are adding vision capacities? Which tools have images which are copyright cleared and used with permission?
Crafting an effective prompt is both an art and a science. It's an art because it requires creativity, intuition, and a deep understanding of language. It's a science because it's grounded in the mechanics of how AI models process and generate responses. // Datacamp
Informed customer/consumer
The library will be interacting with many vendors who purport to have AI features. As discussed further below, these are going to vary enormously, depending on vendor, depth of development and application. Libraries will need to become knowledgeable about claims about AI, functionality, uniqueness - as they are in other areas of procurement. Is the AI component a thin layer over a ChatGPT API or is there more involved? How is retrieval augmented generation being used (a technique to connect the LLM to external data resources, likely to be widely used in library, cultural and scholarly contexts)? And so on. Some libraries may also want to take a position on ethical grounds, in terms of which LLMs are used, for example, which may in turn inform licensing decisions, or advocacy with vendors. Checklists, like the one mentioned above will be valuable.
Advocacy and policy
Policy positions and decisions are important all the way down - whether it is in terms of national policy or institutional decision-making at the college or municipality level. Libraries have a potentially important role in institutional policy making around AI, bringing information policy, ethical and usage concerns to the discussion. This recent article discusses potential library roles and contributions to institutional policy-making.
Principles
Various organizations are developing AI principles or guidelines to help steer their responsible approach to AI. For example, here are the principles adopted by Elsevier. Indeed Gartner advises all organizations to develop what it calls 'lighthouse' principles in this area. In reviewing national AI policies, library dean Leo Lo makes some recommendations for individual libraries in response. These include establishing an AI ethics committee and developing best practices for AI use. It makes sense for libraries to work through some of these issues, and to put in place some explicit ethical and policy frameworks, and a mechanism to review them. Of course, this is also an important area for associations to look at, providing templates or direction as libraries do this work. This is especially so, given the range of capacities available at individual libraries. I was interested to see this work by the World Association of News Publishers as an example. It covers 'intellectual property, transparency, accountability, quality and integrity, fairness, safety, design, and sustainable development.'
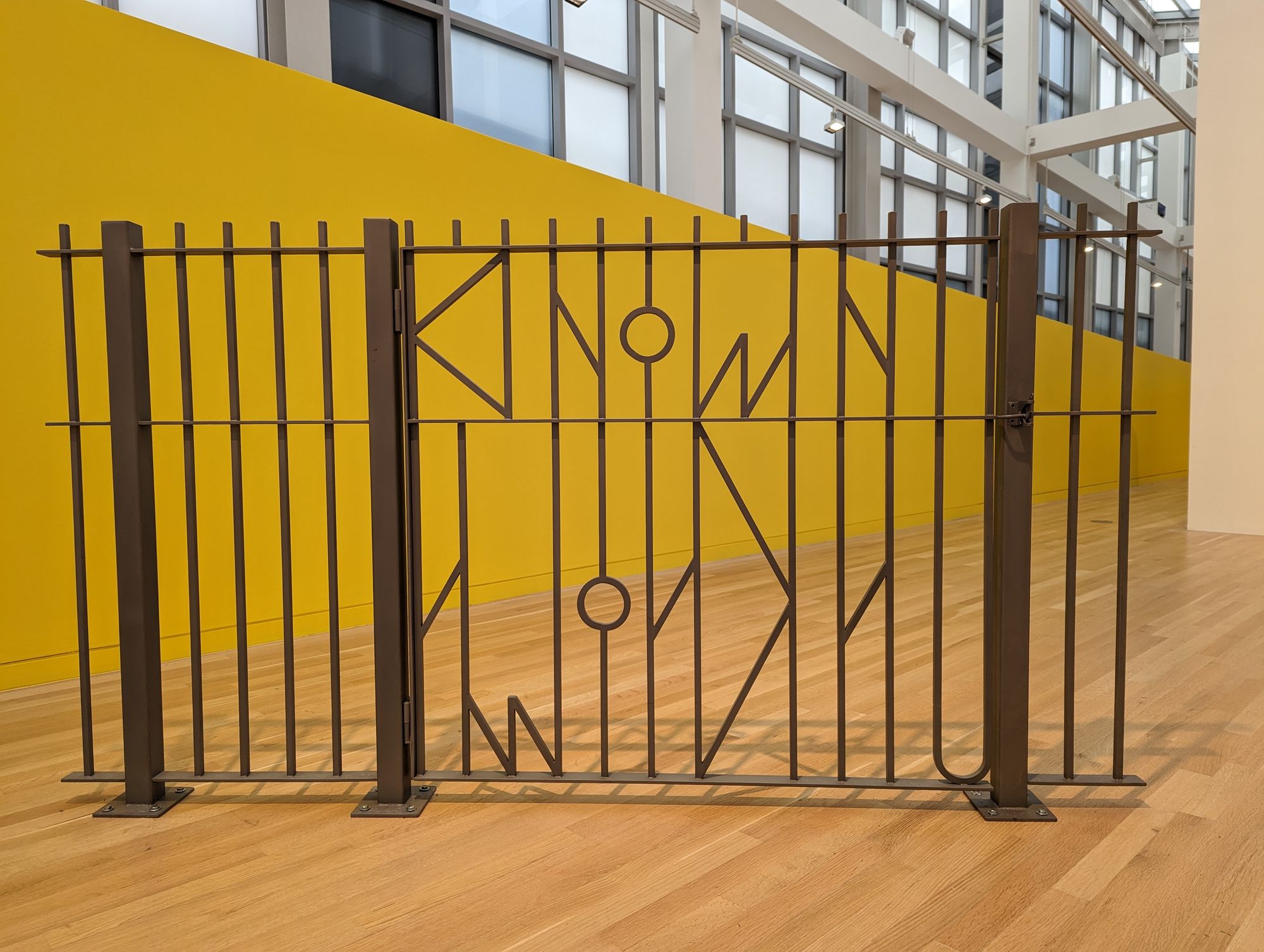
Click for a discussion of social concerns in the AI Background Post
... the Web 2.0 enclosure of creative content, where the originally democratic rhetoric gave way to large monolithic services powered by network effects and winner takes all dynamics.
② AI is extractive and generative
It would appear that some lessons have been learned from the first round of content crawling with Google. And also from the Web 2.0 enclosure of creative content, where the originally democratic rhetoric gave way to large monolithic services powered by network effects and winner takes all dynamics.
Attorney Theresa M. Weisenberger keeps a growing list of copyright litigations involving Generative AI. In many cases, they involve creators unhappy with their creative work being used as part of the training materials for Large Language Models (LLMs). It will be fascinating to watch some of these cases work their way through the courts, and to see how fair use and other issues are interpreted here.

One response is that media companies have also been barring access to OpenAI or other AI companies who want to crawl their content (see the New York Times for example).
At the same time, the AI companies have been arguing that such use constitutes fair use or can be justified in other ways. The Verge highlights some of the arguments made by these companies in submissions to the US Copyright Office. I was interested in Google's argument that 'AI training is just like reading a book.'
Indeed that act of “knowledge harvesting.” to use the Court’s metaphor from Harper & Row, like the act of reading a book ‘and learning the facts and ideas within it, would not only be non-infringing, it would further the very purpose of copyright law. // The Verge
Of course, this time around things are different in an important way. Gen AI tools are being used to generate creative works. Author Jane Friedman wrote about the difficulty she had in getting Amazon and Goodreads to remove books published under her name, but which were produced unknown to her using GenAI. Amazon recently limited the number of books that could be self-published by a single author in a day to three (sic). This was in response to the suspected flood of AI-generated titles.
The Getty has just partnered with Nvidia to release Generative AI by Getty Images, a service trained only on Getty's library of cleared images. A selling point is that this gives users 'full copyright indemnification.' The Getty is in part moving against Stability AI, Midjourney and others who have been using its images without permission.
Academic journals are putting in place recommendations for what type and levels of Gen AI use is acceptable.
So, finally, on the quality question. For BMJ this is so simple. We stand by the quality of what we publish. These tools can create content that looks plausible, and if we get lazy and let things go out into the world without taking responsibility for what we put out there, that is an existential risk to our reputation. That’s why we have now put strict governance around this in place in BMJ. I’d say to that each of us working in the business has a responsibility to educate ourselves around the tools we use. I think you have a responsibility to understand what these tools can do, and to use them responsibly. // Ian Mulvany, CTO, BMJ
More broadly we are now flooded with synthetic text and images, generated by AI. Some of this helpful summarization or extraction, much of it is content marketing, news stories and so on. The potential for defamation, deepfakes and disinformation has grown.
Again the impact on the library is multifaceted.
Copyright and creators. Advice to creators was mentioned above. More generally, the library, and the associations which aggregate library voice and influence, has a role in advocating for the interests of its constituents, balancing their interests as creators with their interests as researchers. For example, I was interested to read this submission to The Copyright Office by the UC Berkeley Library. Of course, few libraries will have the inhouse capacity to produce something like this but it is an example of what an association might produce on their behalf.

Validity and research skills. As also noted above, there are a variety of issues wrapped up in this which belong under the new research skills label. And there are specific tools here also - although we understand that existing detection mechanisms are lacking, and watermarking and other approaches not yet in play. It will be a complex space for a while and we do not yet know what it will settle into.
The cultural record. This broad trend raises fundamental questions for those in scholarly and cultural domains, where transparency, equity and respect for cultural particularity and expression are important. National libraries face interesting questions about what to collect, as do institutional archives. More broadly, for libraries and archives, erosion of social confidence and trust in communication, authorship and creation is complicating, entrusted as they are with the curation of validated knowledge, evidence and memories. This discussion is only beginning.
③ AI and LLMs are not search engines or databases
Apparently, OpenAI itself was surprised at the success of ChatGPT. These technologies after all were already being used in academic and industrial settings for a while. This may account for the way in which it was introduced, where many people assumed it was a form of search engine running against a database of collected material. However, this is a misleading model. It is important to remember that responses are based on inferences about language patterns rather than about what is 'known' to be true, or what is arithmetically correct.
This is how Stephen Wolfram describes how they work:
... it’s just saying things that “sound right” based on what things “sounded like” in its training material. // Writings. Stephen Wolfram
And Isaac Lyman:
It captures more of its essence to say it’s an imitation engine. Having scanned billions of pages of text written by humans, it knows what things a human being is likely to say in response to something. // AI isn't the app, it's the UI
And because of the statistical or probabilistic way in which they work, the patterns they generate may not correspond to reality, leading to so-called hallucinations.
Number 1, reducing hallucination, will be much harder, since hallucination is just LLMs doing their probabilistic thing. // Open challenges in LLM research. Chip Huyen.
So, as well as this plausible but fictitious fabrication (hallucination), responses may, as noted above, reflect historically dominant perspectives about religion, gender or race, or will not be informed by writing about relevant experiences or outlooks that has been suppressed or marginalized in the written record which feeds the training data.
Again, this underlines the important role of understanding how LLMs work so as to offer advice, to select products, and to be informed consumers. The difference between direct interaction with an LLM and interaction with an LLM connected to an external knowledge base or web search in some way is an important consideration here.
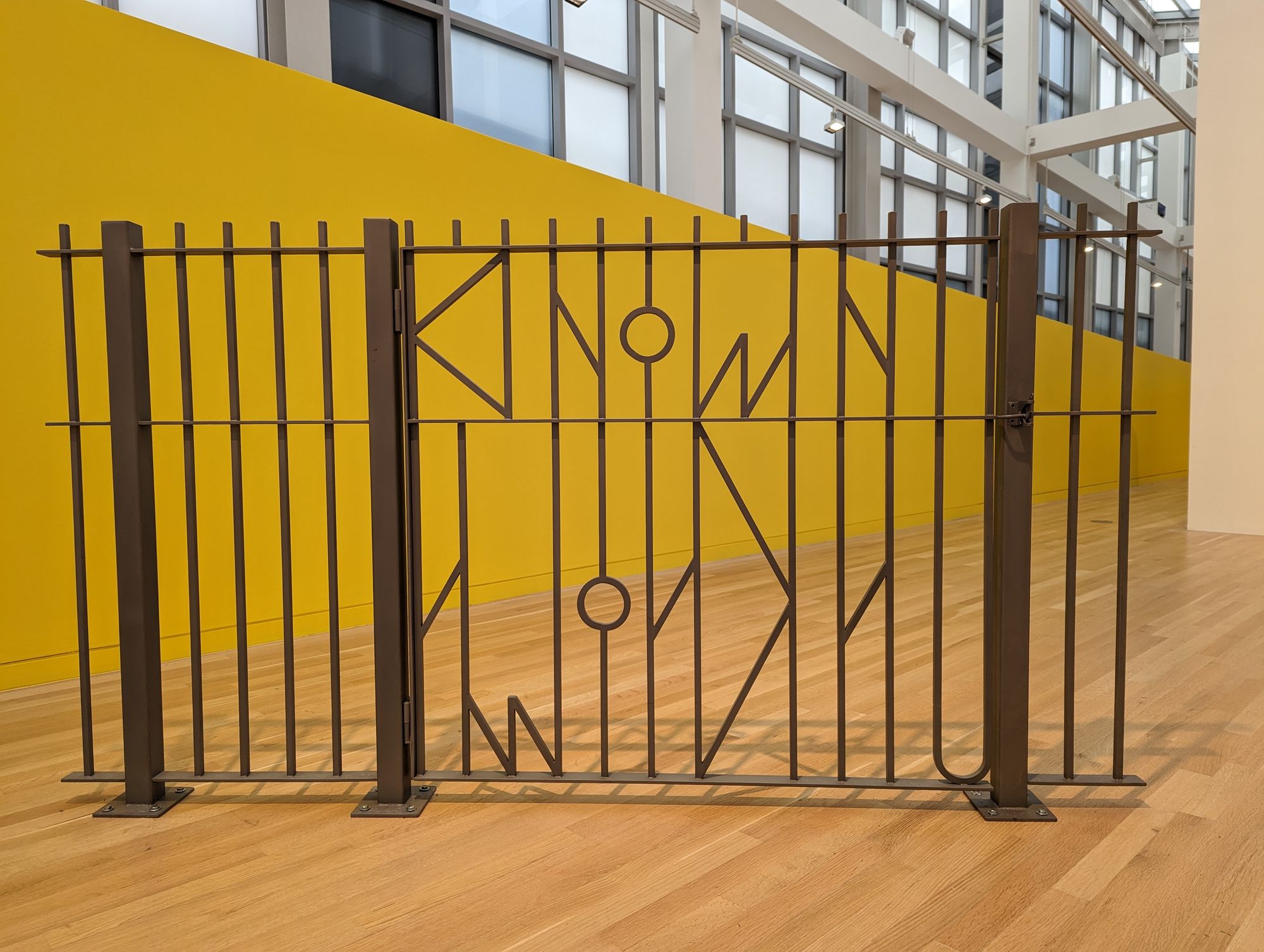
Click for a brief overview of how GenAI works in the AI Background Post
④ AI is appearing differently in different products and services
Historically, libraries relay heavily on their vendors for systems support. Some libraries (or consortia) may have the capacity and interest to experiment themselves, but it is not a large number.
Undoubtedly library vendors, publishers and others are looking closely at their products and services, across a range of issues from enhancement to competitive threat. They will also be looking closely at the cost of any potential development, the increased value it will provide, and how these factors play into pricing and customer willingness to pay. Vendors have very different investment capacities and orientations, so we will see everything from cosmetic features in support of marketing ('we are AI-enabled') to more fundamental re-engineering. This equation will shift as shared infrastructure matures, and third parties make services available through APIs, provide hosting and management services, and so on (see next section).
For varying examples of offers and statements see JSTOR, Ebsco, Talpa (LibraryThing/ProQuest) and OCLC.
Vendors have very different investment capacities and orientations, so we will see everything from cosmetic features in support of marketing ('we are AI-enabled') to more fundamental re-engineering.
In our space, it will be especially interesting to watch what I call the scholarly communication service providers, Elsevier, Digital Science and Clarivate. They each have scale with development capacity, a mature research graph (underlying Scopus, Dimensions and Web of Science, respectively), a wide range of data, publishing and workflow services, as well as connections into scholarly and library communities. They have each made various announcements about AI development [Digital Science/Elsevier/Clarivate]. The announcement of the Dimensions AI Assistant is interesting in that it shows that there will potentially be multiple ways in which applications can be built, and how various components will be orchestrated to achieve results.
“The Dimensions AI Assistant provides users with contextualized synopses using what is known as extractive and abstractive summarization,” says Martin Schmidt, Head of Innovation at Digital Science. “When a user interacts with Dimensions AI Assistant, it scans 33 million articles from the Dimensions database, retrieves and then semantically ranks the top results using semantic embeddings. The abstracts of the top 4 results are then processed by Open AI’s GPT Models API to generate abstractive summaries, and the Dimensions General Science-BERT model is used to provide extractive answers for the top 10 publications,” he explains. // Dimensions
Looking across these offerings one can see that it requires some investigation to see how AI is being used, and what form it takes. Just as in other product areas, it will be important to understand how products work and what they actually do.
⑤ AI is weird
In looking at the rapid spread of AI, I think of a parallel with the mobile technologies that so quickly diffused computational, mapping, and image services into our everyday practices. Those practices, in turn, developed iteratively in unanticipated directions. Think of social media – or even more specifically the selfie – and interaction with communications and travel. And also the baleful interaction with mental wellness. Or think of geolocation services and how we are now accustomed to on-demand mobility, delivery services, navigation, and so on. While it is tempting to extrapolate about the impact of AI, it is good to remember that we cannot yet imagine some of the ways in which it will evolve.
However, there is an important way in which AI is different. Progress is unpredictably uneven.
AI is weird. No one actually knows the full range of capabilities of the most advanced Large Language Models, like GPT-4. No one really knows the best ways to use them, or the conditions under which they fail. There is no instruction manual. On some tasks AI is immensely powerful, and on others it fails completely or subtly. And, unless you use AI a lot, you won’t know which is which. // Ethan Mollick, One Useful Thing
Mollick introduces the Jagged Frontier metaphor to describe this - because capabilities are uneven, they trace a jagged frontier. However, the frontier is often not explicit or visible - some tasks that appear difficult might be readily doable, while others which you might expect machines to do well (basic maths) can be a challenge.
Because of this, it is important to build up some tacit knowledge of how these tools behave, and how they can suprise. Understanding the potential and limitations of AI tools depends on use, and on developing some experiential understanding of behaviours. To explain the difference between Perplexity.ai and ChatGPT one needs to have tried them out. To see the impact of prompt engineering techniques it is helpful to have tried different approaches and seen the quite different results one can achieve. And also to see different results across services.
⑥ AI is cumulative: the infrastructure is getting thicker
Those building or deploying AI applications now have multiple third party infrastructure and platform services which expedite the process and reduce its complexity. A developer may build something end-to-end, but it is likely that they will assemble applications or models building on an increasing rich infrastructure and tool ecosystem. An AI stack is emerging, with capacities potentially provided by multiple players at each level.
For example, at a minimum, an organization may build directly on top of ChatGPT or one of the other services, importing results into their own application.
An organization developing a search application might go further and use an embeddings API from OpenAI or Cohere, or a service from Hugging Face. (Embeddings are numeric representations of words or phrases which provide measures of semantic closeness. For an interesting concrete example read about Cohere's Wikipedia embeddings here.)
An organization wanting to fine-tune an existing LLM also has various options available. Many applications will draw on different sources of data, or LLMs or tools. An organization interested in trying out different LLMs might use the Hugging Face hub. Hugging Face has emerged as a centrally important platform for open source models and related services.
Orchestration frameworks (the open source Langchain, for example, or Fixie, or Semantic Kernel, from Microsoft) are available to allow developers to use LLMs to tie components together in this way.
Hugging Face, Microsoft, Google, Amazon and other platforms have a growing range of tools and services to facilitate cloud management of applications and models.
In this way, organizations may leverage AI capacities in very different ways to get different things done. This may range from a thin application layer over ChatGPT APIs to full stack development and custom LLMs trained on specialist material.
Here are some of the components of special interest to libraries.
Large Language Models
The history of the Internet age suggests that powerful network effects lead to dominant players in various sectors. At the moment, OpenAI and ChatGPT are still dominant, in terms of usage and attention, and seems to be consolidating its lead. Google's initiatives are also important, and Microsoft is partnering with OpenAI (Bing Chat uses models from OpenAI, although it did not initially become as popular as some thought it might, maybe in part because of a not very elegant implementation.) AI startups, Anthropic and Cohere are attracting major investment. Facebook provides significant support for open access LLMs. Will these organizations consolidate their lead, or will others emerge?
Beyond these, there is an evolving and interconnected mix of LLMs, providers and business models. There is strong interest in locally deployable open source models from both commercial and research organizations. And models specialized for particular domains or applications continue to develop. For example, the blog post talking about Dimensions AI Assistant notes that it leverages "the power of the Dimensions large language model, Dimensions General Science-BERT, and Open AI’s GPT models." Forbes has just announced Adelaide, which seems to include a model trained on its own archive.
The tool generates individualized responses to user queries based exclusively on Forbes articles, and is built using Google Cloud Vertex AI Search and Conversation.
We have limited knowledge about what training data, tuning data and other sources are used by the foundation models in general use. In parallel, the transparency, curation and ownership of models (and tuning data) continue to be live issues in the context of research use, alignment with expectations, overall bias, and responsible civic behavior. We also know that some services capture user prompts for fine-tuning, although it may be possible to adjust for privacy.
Tolerances may vary here, as the history of search and social apps have shown, but it can be important to know what particular data is supporting models and applications. Models with more purposefully curated training and tuning data may become more common in scientific and cultural domains and I discuss some examples in the LLM post.
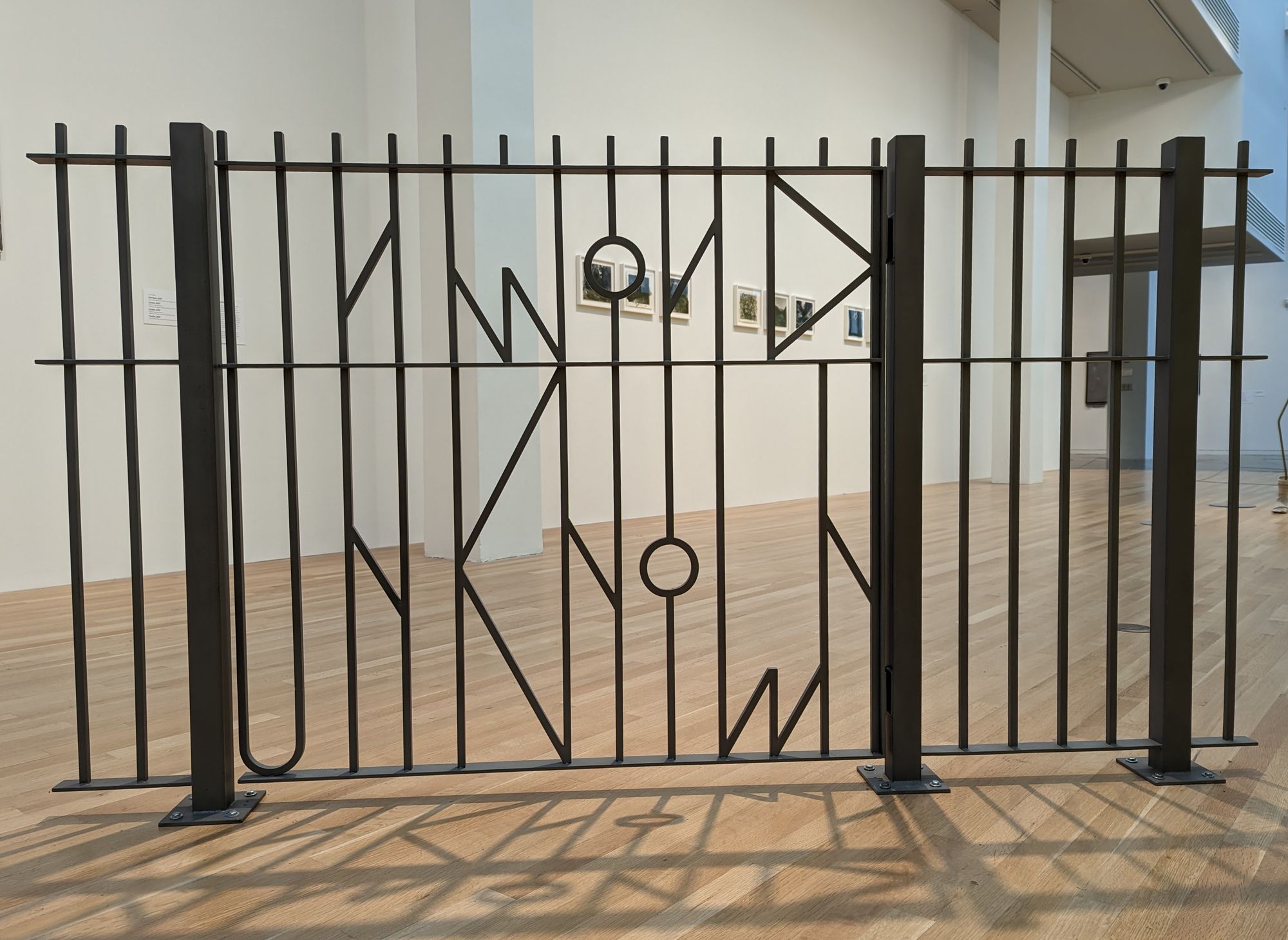
This section draws on the LLM discussion in the two earlier posts. Click here for a discussion of specialist LLMs being developed in scientific and cultural domains
All of this makes it important for libraries to understand the world beyond GPT4, to develop a sense of differences between models, to understand some of the principles behind training, and so on.
For example, Anthropic promotes itself as a builder of 'reliable, interpretable, and steerable AI systems' and has a set of principles for Claude 2, its LLM. These include, for example, principles "Encouraging Consideration of Non-Western Perspectives." An interesting contrast to this is provided by the useful perplexity.ai. It combines Internet search with LLM capabilities to synthesize answers. There is a free version and a subscription version which gives access to GPT4 and Claude 2, as well as experimental access to open source LLMs. However, they have released the open source models without any limits on content or response: "Our models prioritize intelligence, usefulness, and versatility on an array of tasks, without imposing moral judgments or limitations."
In this context, the library also has a role in highlighting LLMs of special interest. This may be where the training data may be of specialist scholarly or cultural interest for example in CORE-GPT, where the largest available corpus of openly accessible articles is being used to train a model [Original description, Progress report]. By using a high-quality, trusted data set in training, the aim is to improve the quality of results and guard against misinformation. Or it may be where care has been taken to only use open or rights-cleared training data. This is the case with the Getty initiative mentioned above (and also, of course, with CORE-GPT).
Finally, as I noted in earlier posts, documentation, evaluation and interpretability are important elements of productive use, and there are interesting intersections here with archival and library practice and skills.
Tools and approaches
Custom Chatbots
We’re rolling out custom versions of ChatGPT that you can create for a specific purpose—called GPTs. GPTs are a new way for anyone to create a tailored version of ChatGPT to be more helpful in their daily life, at specific tasks, at work, or at home—and then share that creation with others. For example, GPTs can help you learn the rules to any board game, help teach your kids math, or design stickers. // OpenAI
This initiative from OpenAI seems a major step forward, significantly reducing the barriers to niche chat-bot creation. It is also a clear signal that OpenAI aims to be a major platform, consolidating its leading position. It will be instructive to explore the utility, quality and variety of GPTs as the store opens. As noted above, one might see a proliferation of custom chatbots, working with highly curated, niche data sources. Of course, whether this is the new app store or an interesting diversion remains to be seen.
Ethan Mollick anticipates significant impact, and is interesting on the potential he sees in his own teaching and writing.
The power here is pretty obvious. I will be creating custom GPTs for every session of the classes I teach. Some will be simulations for students to experience, some will be tutors or mentors, some might even be teammates or assignments. I have been turning my research into GPTs, so that anyone can get advice on how to generate ideas or pitch a business idea by getting feedback from a GPT to which I have given my books as a reference. And I expect this will become a trend in many places, as schools and government agencies and companies build libraries of GPTs that are specialized in solving particular problems in useful ways. // Ethan Mollick, One Useful Thing
Agents and orchestration
The library has special systems and data challenges. There is a reason why interoperability, federation and crosswalks have been so important in library systems contexts.
It is a challenge providing a unified service environment across multiple data resources and systems. Many of these data resources are outside of library control. Libraries also work across many systems, segmented by workflow, data type, source (for example, the ILS, local repositories, external licensed resources, and so on). Library stitching costs are high.
Workflow integrations are also not common and can be fragile, where data moves between processes.
It will be interesting to see how agent and orchestration frameworks are applied to these tasks. It will be interesting to see what libraries and their service partners develop in this space using the text interface and reasoning abilities of LLMs.
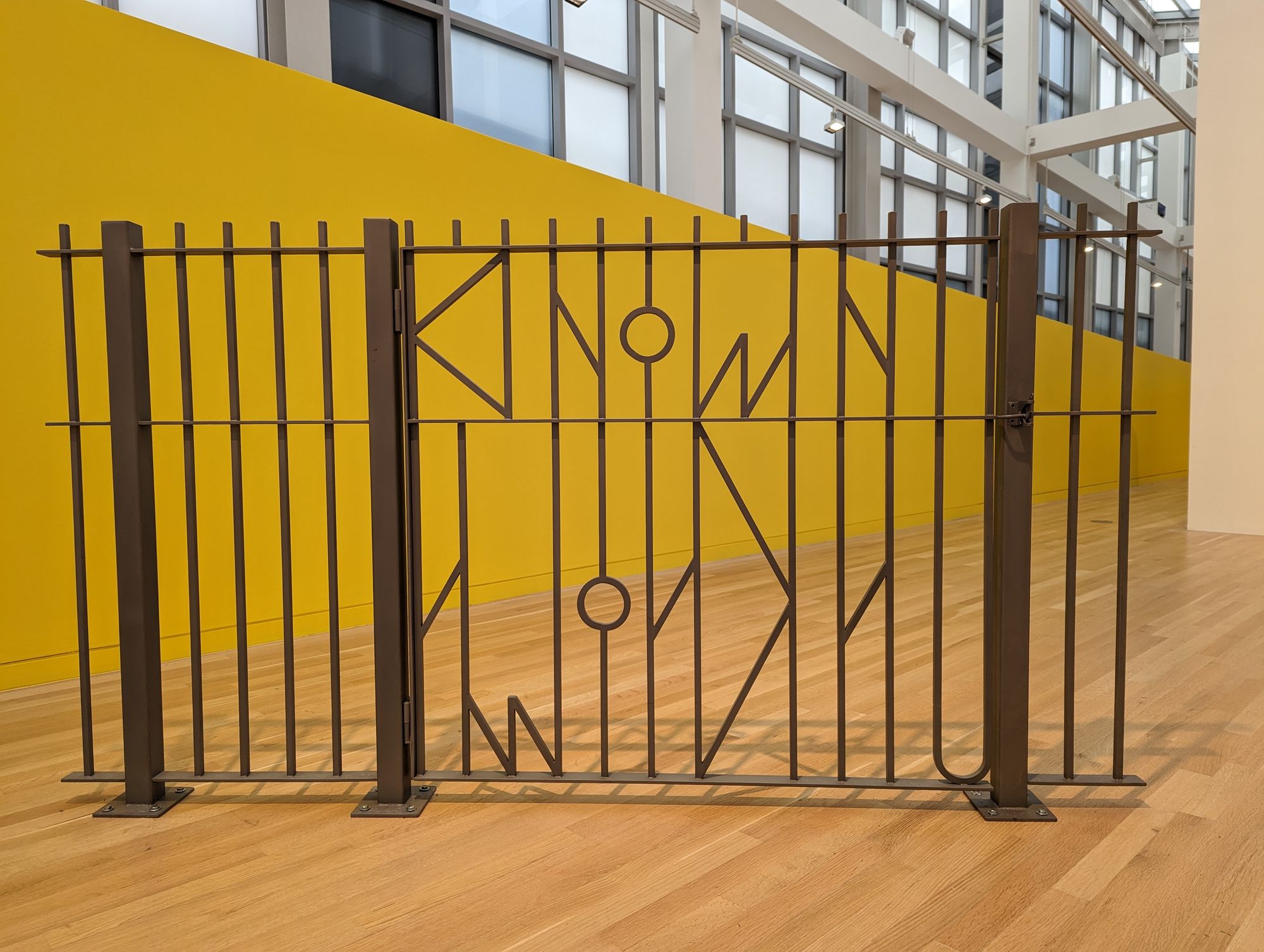
Click for a brief general introduction to agents and orchestration in the AI Background post
Retrieval augmented generation
Based on work by Meta this has emerged as a very important way of extending the capacity of LLMs and grounding their responses in external data resources, whether those are local to an institution or some curated specialist resource.
Retrieval-augmented generation (RAG) is an AI framework for improving the quality of LLM-generated responses by grounding the model on external sources of knowledge to supplement the LLM’s internal representation of information. Implementing RAG in an LLM-based question answering system has two main benefits: It ensures that the model has access to the most current, reliable facts, and that users have access to the model’s sources, ensuring that its claims can be checked for accuracy and ultimately trusted. // IBM
This model, however implemented, will be popular among services licensed or used by libraries to combine the text processing abilities of the LLM with curated data resources. The examples from Digital Science, Clarivate and Elsevier above are indicative of direction here.
Embeddings and vector databases
I mentioned embeddings earlier. Embedding APIs are now available from several organizations. Vector databases are an emerging technology which is important for managing embeddings which in turn will drive new discovery and related services. It should be noted that embeddings will exhibit the same prejudicial or stereotyping behaviors as the LLMs to which they are related (doctors are men, nurses are women).
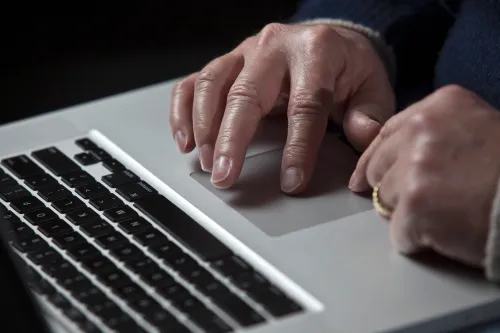
Prompt engineering
Prompt engineering was discussed in general terms above. It uses one of those 'weird' properties of LLMs: in-context learning. It is possible to modify the behavior of LLMs based on what is provided as input, without altering the underlying model or training data. For this reason it is an area of intense investigation, guidance and commentary. Tools and preprocessing techniques are being used to manage, refine, and adapt prompts. LLMs themselves are being used to refine prompts, or to prepare prompts for other services. And again, this can be an important element of partial mitigation strategies in the context of hallucination or harmful outputs.
Co-pilot
Github and Microsoft popularised the co-pilot metaphor ... where a human activity is 'assisted' by an AI application. Any individual application will calibrate the balance of assistance and the type of interaction and control. The model is now familiar in coding and search, and Microsoft is rolling out co-pilot assistance in Office applications. These are increasingly common: examples are Sidekick in Shopify or Genmo Chat for assisting with image generation.
In a library context, metadata creation and cataloging immediately come to mind as areas where co-pilot assistance could help, working to inform human judgement with additional data or tying other tools into the workflow. Reference also. The model is interesting because applications can be designed to assist human judgement and decisions rather than substitute for them.
Make, bake or take
Very few libraries will have the capacity to develop or 'make' AI applications from scratch. Although there will certainly be some experiment and grant-funded activity. Many libraries will adopt, or 'take', ready-made solutions, from third-party services of various types. However, the ready availability of component APIs and platforms means that some libraries may explore 'baking', where they combine components to build some local functionality. In practice, 'baking' is the approach taken by many library vendors as they build products, based on APIs and LLMs available in various ways.
In practice, 'baking' is the approach taken by many library vendors as they build products, based on APIs and LLMs available in various ways.
⑦ AI is complicated: policy and governance
The discussion around openness is taking place against the backdrop of an impassioned debate about how we navigate governance and (existential) risk. As we forecast in last year’s report, safety has shed its status as the unloved cousin of the AI research world and took center-stage for the first time. As a result, governments and regulators around the world are beginning to sit up and take notice. // Welcome to State of AI Report 2023
It is certainly center stage as I write this. I noted the US White House Executive Order above. And the UK Government convened a high level international AI summit to confer on issues. Leo Lo usefully reviews some national AI policies from around the world, although as work progresses here this will date.
The major AI players have also been talking about self governance or industry practices. For example, OpenAI, Google, Microsoft and Anthropic have jointly set up the Frontier Model Forum, to coordinate around safety issues.
The impact of regulation varies across jurisdictions with different political cultures and views on the matter (as seen, for example, historically in the case of the European General Data Protection Regulation (GDPR)). The balance between industry and government may be a key point of divergence, with concerns about regulatory capture in some contexts arising from the influence of large incumbent interests on regulatory decisions. While libraries generally support greater regulation, there are also well-known side-effects, especially if the cost or complexity of compliance is significant. This aspect can pose challenges for open-source or non-commercial players, for example, who may struggle to comply with complex regulatory frameworks and compete with well-established industry players. And there is an argument also that it is too early, that not enough is known about impacts or what actually should be regulated.
As we know, universities are developing guidance and frameworks around pedagogical and research use. Organizations are thinking about the implications of use in HR and elsewhere. Scholarly publishers are putting in place policies about acceptable AI contribution. And decisions over the next while about copyright, fair use, and related issues will gradually put in place a richer framework, although it may vary from country to country and take some time.
While the library can play an important role within their community in promoting appropriate guidelines around AI use, there is also the broader context of library influence on public policy.
Traditionally, the library community has scaled its influence, or voice, through various associations: ALA, ARL or ULC in the US, for example, or LIBER and IFLA internationally. These organizations have taken up policy issues, and lobbied at state, national or international levels. Copyright has been a major historical focus, sharpened in recent years in a digital context.
Again, we can expect to see these organizations carry a library interest (which is a public interest) to decision- and policy-makers. It is early days but I hope these organizations are building an agenda for attention. Areas of interest might include transparency of models and disclosure of practices (see Tim O'Reilly's remarks here), the near-term social costs of the models (for example, toxic effects on hidden labor), documentation, balancing the rights of creators and researchers, and beginning the discussion about LLMs, synthesised creations and the cultural record.
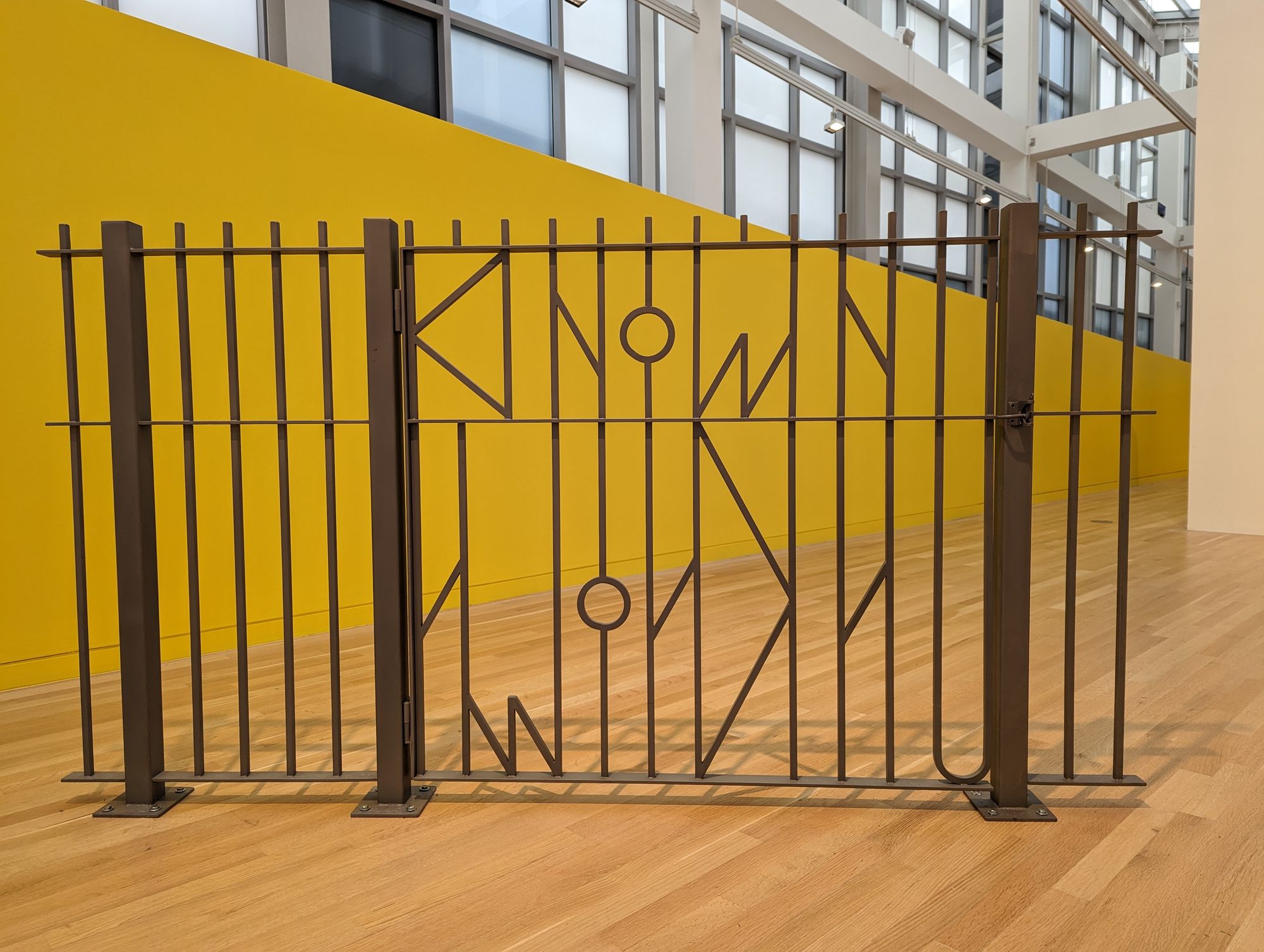
Click here for some discussion of regulation in the AI Background Post
Conclusion
So, AI is becoming mainstream, unevenly, and is at once productive and problematic. It is being introduced into products and services, in different ways. Library users are engaging with AI tools and techniques, also very unevenly.
This means that the library discussion is necessarily moving towards forms of engagement, cautiously, as questions come up in terms of user support and awareness, procurement, staff preparedness and medium-range planning.
I think the University of Leeds has provided an admirable and useful example as it commissioned some work which explored the views and expectations of library stakeholders.

Perhaps the findings are not very remarkable. The approach (library stakeholder interviews) naturally produces an evolutionary perspective, based on current expectations. It illustrates how thoughtful application of AI approaches may be productive, and acknowledges the problematic. It will be interesting to consider the library at Leeds in, say, 5 years, and to compare it with directions outlined here.
By then, maybe, AI will have become more routine and addressed within general processes. There may also be completely unanticipated outcomes. For now, extra care and special attention are certainly warranted.
Picture: I took the feature photo on the way into work.
Acknowledgements: I am very grateful to Nicole Hennig, Lynne M. Thomas and Melanie Walsh for generously making helpful suggestions on an earlier draft.